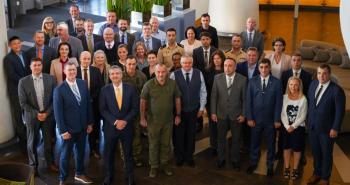
PfP Consortium ADL Working Group
Jefferson Institute President Dr. Aaron Presnall is co-chair of the NATO Partnership for Peace Consortium’s Advanced Distributed Learning (ADL) Working Group, spearheading multinational cooperation to innovate eLearning and build interoperable, resilient, and agile military education and training capabilities.